Low-friction data structures are those that: 1) are self-describing, 2) directly represent that which they model, and 3) do not require transformation between operations and analytics. Our graph-throughout approach to Hybrid Transactional / Analytic Processing system development encourages a direct connection between business and IT where data model creation can be collaborative.
Once the discrete, well-bounded RDF data model is designed, the backend system is generated, ready for: 1) behavioral augmentation where necessary, 2) test, and 3) deploy. Monolith and command-and-control are out, well-bounded microservice, distributed, and peer-to-peer are in.
This low-code approach helps to address the: 1) human resource impact of adopting new technology, 2) communication overhead of translating business concerns to production enterprise software, 3) adaptability of systems to business need, and 4) explicit connection between operational and analytic systems.
In contrast to relational and columnar data management which rely on implicit relationships embedded in SQL, Temporal Linked Data® (TLD) makes an explicit connection between temporal data aggregates.
Likewise, where Event Stores keep all data domain events together, TLD keeps all temporal data alongside the aggregate to which it belongs.
Explicit connections are both hard and soft, technical and social. Hybrid Transactional / Analytic Processing by way of TLD explicitly connects:
- Temporal data aggregates,
- Operational data with analytic data for realtime analysis,
- Business and IT personnel, and
- Represents the shortest path between business concept and production deployment.
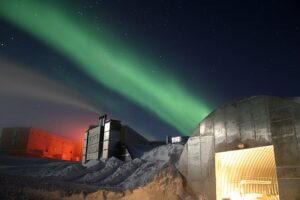
Amundsen Scott South Pole Station, a low-friction location to view the night sky (NSF Public Domain Image)